Documentation / Manuel développeur
Modules disponibles
Calibration,  DataServer,  Launcher,  MetaModelOptim,  Modeler,  Optimizer,  ReLauncher,  Reliability,  ReOptimizer,  Sampler,  Sensitivity,  UncertModeler,  XmlProblem,  ![]() |
Uranie / Modeler v4.9.0
|
#include <TANNModeler.h>
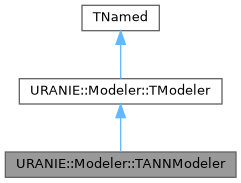
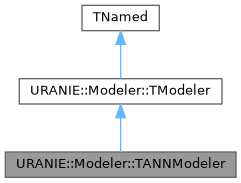
Public Types | |
enum | EProblem { kRegression , kClassification } |
enum | ENorm { kCR , kMinusOneOne , kZeroOne } |
Public Member Functions | |
Constructor and Destructor | |
TANNModeler (URANIE::DataServer::TDataServer *tds, TString architecture, Double_t dratio=0.80, Option_t *option="") | |
Constructor with a dataserver. | |
virtual | ~TANNModeler () |
Default destructor. | |
Setter and Getter function | |
Int_t | getNInput () |
Gets the number of input. | |
Int_t | getNHidden () |
Gets the number of hidden neurons. | |
Int_t | getNOutput () |
Gets the number of output. | |
Int_t | getNAPP () |
Gets the number of pure training patterns ("APPrentissage") | |
Int_t | getNTest () |
Gets the number of pure training patterns ("APPrentissage") | |
void | setWeights (ColumnVector weights) |
Sets the weigts. | |
ColumnVector | getWeights () |
Double_t | getWeight (Int_t ind) |
Returns the weight givent by the index. | |
Double_t | getMinValue (Int_t ind) |
Double_t | getMaxValue (Int_t ind) |
Double_t * | getdval () |
void | setValue (Int_t ind, Int_t &ncrt, Double_t *xy, Bool_t normalize=true) |
void | setDataSet (URANIE::DataServer::TPatternsEventList *tel) |
void | setMix (Bool_t bmix=kTRUE) |
void | setFcnTol (Double_t dprec) |
Double_t | getFcnTol () |
void | setNormalization (ENorm n) |
Sets the normalisation. | |
ENorm | getNormalization () |
Get the normalisation. | |
EProblem | getProblem () |
Get the problem type. | |
void | setSeed (Int_t ind=0) |
Init the seed. | |
Int_t | getSeed () |
Returns the seed value. | |
const char * | getInputName (Int_t i) |
Return the name of input attributes indexed by i. | |
const char * | getOutputName () |
Get the attribute name of the output. | |
Training | |
void | init () |
void | randomizeWeights () |
randomize the weights | |
void | train (Int_t niter=10, Int_t nInit=10, Option_t *option="text", Bool_t useGPU=false) |
Double_t | getEQM (Option_t *option="") |
Gets the EQM. | |
Double_t | getWeightDecay () |
Get the weight decay parameter. | |
void | saveIfBetter (const ColumnVector &weights, Double_t eqm_app, Double_t eqm_tst) |
Save a vector of weight if the RMS on the test database is better than what we currently have. | |
Exports function | |
The exports function to save an Artificial Neural Networks in an external format
The user fonction is the exportFunction method. tann->exportFunction("c++", "uranie_ann_flowrate.C","ANNflowrate");
tann->exportFunction("fortran", "uranie_ann_flowrate.f","ANNflowrate");
tann->exportFunction("pmml", sPMMLFile, sANNName,"new");
tann->exportFunction("C++,fortran,pmml", "uranie_ann_flowrate","ANNflowrate");
| |
void | exportModelCplusplus (const char *file="", const char *name="", Option_t *option="") const |
The only user interface method. | |
void | exportModelFortran (std::ofstream *sourcefile) const |
void | exportModelPython (std::ofstream *sourcefile) const |
Export the model in Python langage in a file (not yet implemented) | |
Printing Log | |
virtual void | printLog (Option_t *option="") |
![]() | |
TModeler (URANIE::DataServer::TDataServer *tds, TString architecture, Option_t *option="") | |
Constructor with a dataserver. | |
TModeler (URANIE::DataServer::TDataServer *tds, const char *varexpinput, const char *varexpoutput, Option_t *option="") | |
Default constructor with input and output attributes. | |
virtual | ~TModeler () |
Default destructor. | |
TVectorD | getParameters () |
Return the parameter vector of the model. | |
Double_t | getR2 () const |
Return the R2 quality. | |
const char * | getInputName (Int_t i) const |
Return the name of input attributes. | |
const char * | getOutputName (Int_t i=0) |
Return the name of output attribute. | |
Int_t | getNInput () |
Gets the number of input. | |
Bool_t | getIntercept () |
Gets the boolean attribut _bIntercept. | |
void | setDrawProgressBar (Bool_t bbool=kTRUE) |
Set the "draw progress bar" flag. | |
Bool_t | getDrawProgressBar () |
Get the "draw progress bar" flag. | |
void | train (Option_t *option="text") |
virtual void | exportFunction (const char *lang, const char *file="", const char *name="", Option_t *option="") |
Export the model in an external file with a specified langage. | |
virtual void | exportModelCplusplus (std::ofstream *sourcefile) const =0 |
Export the model in C++ langage in a file. | |
virtual void | exportModelPMML (const char *file="", const char *name="", Option_t *option="") const =0 |
Export the model in a PMML file. | |
void | setLog () |
void | unsetLog () |
void | changeLog () |
Bool_t | getLog () |
Protected Attributes | |
Int_t | _nSeed |
Private Attributes | |
Int_t | _nInput |
! Seed for the random weight initialization | |
Int_t | _nHidden |
! The number of inputs | |
Int_t | _nHiddenLayer |
! The number of hidden neurons | |
Int_t | _nOutput |
! the number of hidden layer | |
Int_t | _nNeurons |
! The number of outputs | |
Int_t | _nApp |
! The number of neurons | |
Int_t | _nTest |
! Number of samples in the training set dedicated to back-propagation | |
Double_t | _dLearn |
! Number of samples in the training set dedicated to validation | |
Double_t | _dTest |
! The learning quality | |
Double_t | _dWeightDecay |
! The test quality | |
URANIE::DataServer::TPatternsEventList * | _elAll |
! The weight decay parameter - when used, favorize a smoother ANN | |
Bool_t | _bMix |
! The mix list of patterns | |
TString | _sArchi |
! True if data set must be mixed | |
TString | _sInput |
! The architecture, e.g. "x:y:z,3,yhat" specifying inputs, number of hidden neurons, and output | |
TString | _sHidden |
TString | _sOutput |
Int_t | _nWeight |
ColumnVector | _vecWeights |
! The number of weights | |
TempSolution * | _tmpSolution |
ColumnVector | _vecMinValues |
ColumnVector | _vecMaxValues |
! Min values, used for normalization | |
TList * | _listOfAttributes |
! Max values, used for normalization | |
Parameters of algorithm | |
Double_t | _dFcnTol |
ENorm | _nNormType |
! The function tolerance for the trust region algorithm | |
EProblem | _nProlemType |
! The normalized for input and output | |
Double_t * | _dval |
! The type of problem (Regression, Classification) | |
static const int | OPTIM_MAX_ITER = 10000 |
static const int | OPTIM_MAX_FEVAL = 50000 |
Bool_t | _blog |
Boolean for edit the log. | |
URANIE::DataServer::TDataServer * | _tds |
UMLP * | _UMLP |
Pointeur vers un TDS. | |
Additional Inherited Members | |
![]() | |
Bool_t | _blog |
Boolean for edit the log. | |
Bool_t | _bdrawProgressBar |
Boolean to know if the progress bar has to be drawn. | |
Bool_t | _bStoreYHat |
Boolean to specify if we add the \hat{} attribute in the TDS [default kTRUE]. | |
URANIE::DataServer::TDataServer * | _tds |
Int_t | _nS |
Pointer to a TDS. | |
TString | _sInputAttributes |
The string of input attributes (separated by the character ":". | |
TString | _sOutputAttributes |
The string of output attributes (separated by the character ":". | |
TString | _sIntermediateArchitecture |
The intermediate (between the first and last character ",") information. | |
TList * | _listOfInputAttributes |
The list of input Leaf. | |
TList * | _listOfOutputAttributes |
The list of output Leaf. | |
Int_t | _nX |
The number of input attributes. | |
Int_t | _nY |
The number of output attributes. | |
TString | _sFileName |
The name of the file when export the model without extension. | |
TString | _sFunctionName |
The name of the function when export the model. | |
TVectorD | _parameterValues |
The list of parameters. | |
Double_t | _dr2 |
The R2 quality. | |
Bool_t | _bIntercept |
For certain models, add the intercept (Default is TRUE) | |
Member Enumeration Documentation
◆ ENorm
◆ EProblem
Constructor & Destructor Documentation
◆ TANNModeler()
URANIE::Modeler::TANNModeler::TANNModeler | ( | URANIE::DataServer::TDataServer * | tds, |
TString | architecture, | ||
Double_t | dratio = 0.80 , |
||
Option_t * | option = "" |
||
) |
Constructor with a dataserver.
Referenced by ClassImp().
◆ ~TANNModeler()
|
virtual |
Default destructor.
Referenced by ClassImp().
Member Function Documentation
◆ exportModelCplusplus()
void URANIE::Modeler::TANNModeler::exportModelCplusplus | ( | const char * | file = "" , |
const char * | name = "" , |
||
Option_t * | option = "" |
||
) | const |
The only user interface method.
- Parameters
-
lang (const char*) the keyword for the external format [c++|fortran|pmml] file (const char*) the filename. If empty, it is the name of the TDS with the good extension.[""] name (const char*) name of the function. option (const char*) option parameter. - NEW : create a new PMML file
- UPDATE : Write the current ANN in the PMML file and if already exist an ANN in the PMML file, erase it.
- BACKUP : Write the current ANN in the PMML file and if already exist ANNs in the PMML file with modelname "name_I", rename the old with "name_I+1" Export the ANN model in a ROOT macro file
Referenced by ClassImp().
◆ exportModelFortran()
|
virtual |
- Todo:
- Write this method as a TModeler method / void exportModelCplusplus(std::ofstream * sourcefile) const; { cerr << " --- Method TANNModeler::exportModelCplusplus(ofstream * sourcefile) not yet implemented" << endl; cerr << " --- Then using the method TANNModeler::exportModelCplusplus(file, name, option)" << endl; } ;
! Export the TANNModeler object in a PMML File void exportModelPMML(const char* file = "", const char* name = "", Option_t option = "") const; ! Export the ANN model in a Fortran file void exportModelFortran(const char file, const char* name, Option_t option = "") ; /!
Implements URANIE::Modeler::TModeler.
Referenced by ClassImp().
◆ exportModelPython()
|
inlinevirtual |
Export the model in Python langage in a file (not yet implemented)
- Warning
- Don't use this method. Use the main method TModeler::exportFunction.
- Parameters
-
sourcefile (ofstream *) the pointer to the file to export the modeler.
Implements URANIE::Modeler::TModeler.
◆ getdval()
|
inline |
References _dval.
Referenced by ClassImp().
◆ getEQM()
Double_t URANIE::Modeler::TANNModeler::getEQM | ( | Option_t * | option = "" | ) |
Gets the EQM.
- Parameters
-
option ["learn|test"] give the Mean Square Error (MSE ) on the learn database ("learn") or on the test database ("test").
Referenced by ClassImp().
◆ getFcnTol()
|
inline |
References _dFcnTol.
Referenced by ClassImp().
◆ getInputName()
|
inline |
Return the name of input attributes indexed by i.
References _listOfAttributes.
◆ getMaxValue()
|
inline |
References _vecMaxValues.
Referenced by ClassImp().
◆ getMinValue()
|
inline |
References _vecMinValues.
Referenced by ClassImp().
◆ getNAPP()
|
inline |
Gets the number of pure training patterns ("APPrentissage")
References _nApp.
Referenced by ClassImp().
◆ getNHidden()
|
inline |
◆ getNInput()
|
inline |
◆ getNormalization()
|
inline |
◆ getNOutput()
|
inline |
◆ getNTest()
|
inline |
Gets the number of pure training patterns ("APPrentissage")
References _nTest.
Referenced by ClassImp().
◆ getOutputName()
|
inline |
Get the attribute name of the output.
References _sOutput.
◆ getProblem()
|
inline |
◆ getSeed()
|
inline |
Returns the seed value.
References _nSeed.
◆ getWeight()
|
inline |
Returns the weight givent by the index.
the index begins to 0 and ends to nW-1
References _vecWeights.
◆ getWeightDecay()
|
inline |
◆ getWeights()
|
inline |
References _vecWeights.
Referenced by ClassImp().
◆ init()
void URANIE::Modeler::TANNModeler::init | ( | ) |
Referenced by ClassImp().
◆ printLog()
|
virtual |
Reimplemented from URANIE::Modeler::TModeler.
Referenced by ClassImp().
◆ randomizeWeights()
void URANIE::Modeler::TANNModeler::randomizeWeights | ( | ) |
randomize the weights
Referenced by ClassImp().
◆ saveIfBetter()
void URANIE::Modeler::TANNModeler::saveIfBetter | ( | const ColumnVector & | weights, |
Double_t | eqm_app, | ||
Double_t | eqm_tst | ||
) |
Save a vector of weight if the RMS on the test database is better than what we currently have.
Referenced by ClassImp().
◆ setDataSet()
|
inline |
References _elAll.
◆ setFcnTol()
|
inline |
References _dFcnTol.
◆ setMix()
|
inline |
References _bMix.
◆ setNormalization()
void URANIE::Modeler::TANNModeler::setNormalization | ( | ENorm | n | ) |
Sets the normalisation.
Referenced by ClassImp().
◆ setSeed()
void URANIE::Modeler::TANNModeler::setSeed | ( | Int_t | ind = 0 | ) |
Init the seed.
If the value is zero, the seed is initialized with the hour in milliseconds
- Parameters
-
ind (Int_t) the seed value
Referenced by ClassImp().
◆ setValue()
void URANIE::Modeler::TANNModeler::setValue | ( | Int_t | ind, |
Int_t & | ncrt, | ||
Double_t * | xy, | ||
Bool_t | normalize = true |
||
) |
Referenced by ClassImp().
◆ setWeights()
|
inline |
◆ train()
void URANIE::Modeler::TANNModeler::train | ( | Int_t | niter = 10 , |
Int_t | nInit = 10 , |
||
Option_t * | option = "text" , |
||
Bool_t | useGPU = false |
||
) |
Launch the training of the ANN. This potentially uses the GPU if available.
- Parameters
-
niter number of times (a random permutation of) the test base will be presented for training nInit number of times the ANN is trained (from random start weights) with a given permutation of the test database
Referenced by ClassImp().
Member Data Documentation
◆ _blog
Bool_t URANIE::Modeler::TANNModeler::_blog |
Boolean for edit the log.
Referenced by ClassImp().
◆ _bMix
|
private |
! The mix list of patterns
Referenced by ClassImp(), and setMix().
◆ _dFcnTol
|
private |
Referenced by ClassImp(), getFcnTol(), and setFcnTol().
◆ _dLearn
|
private |
! Number of samples in the training set dedicated to validation
Referenced by ClassImp().
◆ _dTest
|
private |
! The learning quality
Referenced by ClassImp().
◆ _dval
|
private |
! The type of problem (Regression, Classification)
Temporary array used during training
Referenced by ClassImp(), and getdval().
◆ _dWeightDecay
|
private |
! The test quality
Referenced by ClassImp(), and getWeightDecay().
◆ _elAll
|
private |
! The weight decay parameter - when used, favorize a smoother ANN
Referenced by ClassImp(), and setDataSet().
◆ _listOfAttributes
|
private |
! Max values, used for normalization
Referenced by ClassImp(), and getInputName().
◆ _nApp
|
private |
! The number of neurons
Referenced by ClassImp(), and getNAPP().
◆ _nHidden
|
private |
! The number of inputs
Referenced by ClassImp(), and getNHidden().
◆ _nHiddenLayer
|
private |
! The number of hidden neurons
Referenced by ClassImp().
◆ _nInput
|
private |
! Seed for the random weight initialization
Referenced by ClassImp(), and getNInput().
◆ _nNeurons
|
private |
! The number of outputs
Referenced by ClassImp().
◆ _nNormType
|
private |
! The function tolerance for the trust region algorithm
Referenced by ClassImp(), and getNormalization().
◆ _nOutput
|
private |
! the number of hidden layer
Referenced by ClassImp(), and getNOutput().
◆ _nProlemType
|
private |
! The normalized for input and output
Referenced by ClassImp(), and getProblem().
◆ _nSeed
|
protected |
Referenced by ClassImp(), and getSeed().
◆ _nTest
|
private |
! Number of samples in the training set dedicated to back-propagation
Referenced by ClassImp(), and getNTest().
◆ _nWeight
|
private |
Referenced by ClassImp().
◆ _sArchi
|
private |
! True if data set must be mixed
Referenced by ClassImp().
◆ _sHidden
|
private |
Referenced by ClassImp().
◆ _sInput
|
private |
! The architecture, e.g. "x:y:z,3,yhat" specifying inputs, number of hidden neurons, and output
Referenced by ClassImp().
◆ _sOutput
|
private |
Referenced by ClassImp(), and getOutputName().
◆ _tds
URANIE::DataServer::TDataServer* URANIE::Modeler::TANNModeler::_tds |
Referenced by ClassImp().
◆ _tmpSolution
|
private |
Referenced by ClassImp().
◆ _UMLP
UMLP* URANIE::Modeler::TANNModeler::_UMLP |
Pointeur vers un TDS.
Referenced by ClassImp().
◆ _vecMaxValues
|
private |
! Min values, used for normalization
Referenced by ClassImp(), and getMaxValue().
◆ _vecMinValues
|
private |
Referenced by ClassImp(), and getMinValue().
◆ _vecWeights
|
private |
! The number of weights
! The vector of weights, stored like this: [a][b(1) b(2) b(3).... b(_nInput+2)][c(1) c(2) c(3).... c(_nInput+2)][ ... ] a: scalar value giving the weight of the output biais (link betw. single output and biais neuron in hidden layer) b, c, d, ...: _nHidden blocks of (_nInput+2) scalars representing: b(1): weight link between second hidden neuron and output (c(1) for the 3rd hidden neuron, d(1) for the 4th, etc ...) b(2): weight between biais input neuron and second hidden neuron b(2+i): weight between i-th (real) input neuron and second hidden neuron See impl. of UMPL::evaluateInput().
Referenced by ClassImp(), getWeight(), getWeights(), and setWeights().
◆ OPTIM_MAX_FEVAL
|
staticprivate |
Referenced by ClassImp().
◆ OPTIM_MAX_ITER
|
staticprivate |
Referenced by ClassImp().