The LIAD team
Aurore LOMET
Engineer-researcherAurore Lomet is a researcher in causal discovery (artificial intelligence, machine learning, and time series analysis). Her PhD, obtained from the University of Technology of Compiègne (UTC), focused on Model Selection for Co-Clustering of Continuous Data, under the supervision of Gérard Govaert and Yves Grandvalet. In this context, her PhD aimed to propose objective criteria for comparing multiple co-clustering methods for continuous data, considering the non-i.i.d. (non-independent and identically distributed) case.
After her PhD, Aurore joined CEA as a post-doctoral researcher, where she developed statistical models and clustering techniques to predict hot water consumption. This work continued to explore the challenges associated with non-i.i.d. data, particularly in the context of hot water consumption, where the collected data exhibits dependencies and temporal structures.
Subsequently, she was employed as a research engineer in applied mathematics and statistical modeling. Her work continues to address non-i.i.d. data, a special case in statistics where "classical" properties such as asymptotic properties are not always defined. In this scope, she has worked on various projects, including: Causality with Bayesian graphs for anomaly detection in an industrial setting based on sensor measurements, Trusted AI with uncertainty to predict parking time, Nuclide detection using deep and transfer learning, and Interpretable AI for border security.
In 2023, Aurore joined the LIAD laboratory, where she focuses her work on causal discovery, particularly for time series, and the quantification of uncertainties. In addition to her research and project management activities, she supervises interns, doctoral students, and post-doctoral researchers, and also teaches introductory supervised AI courses at Centrale-Supelec.
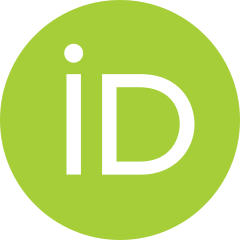
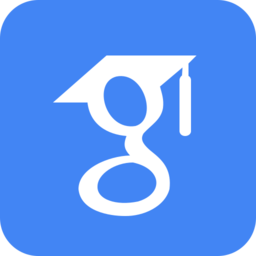
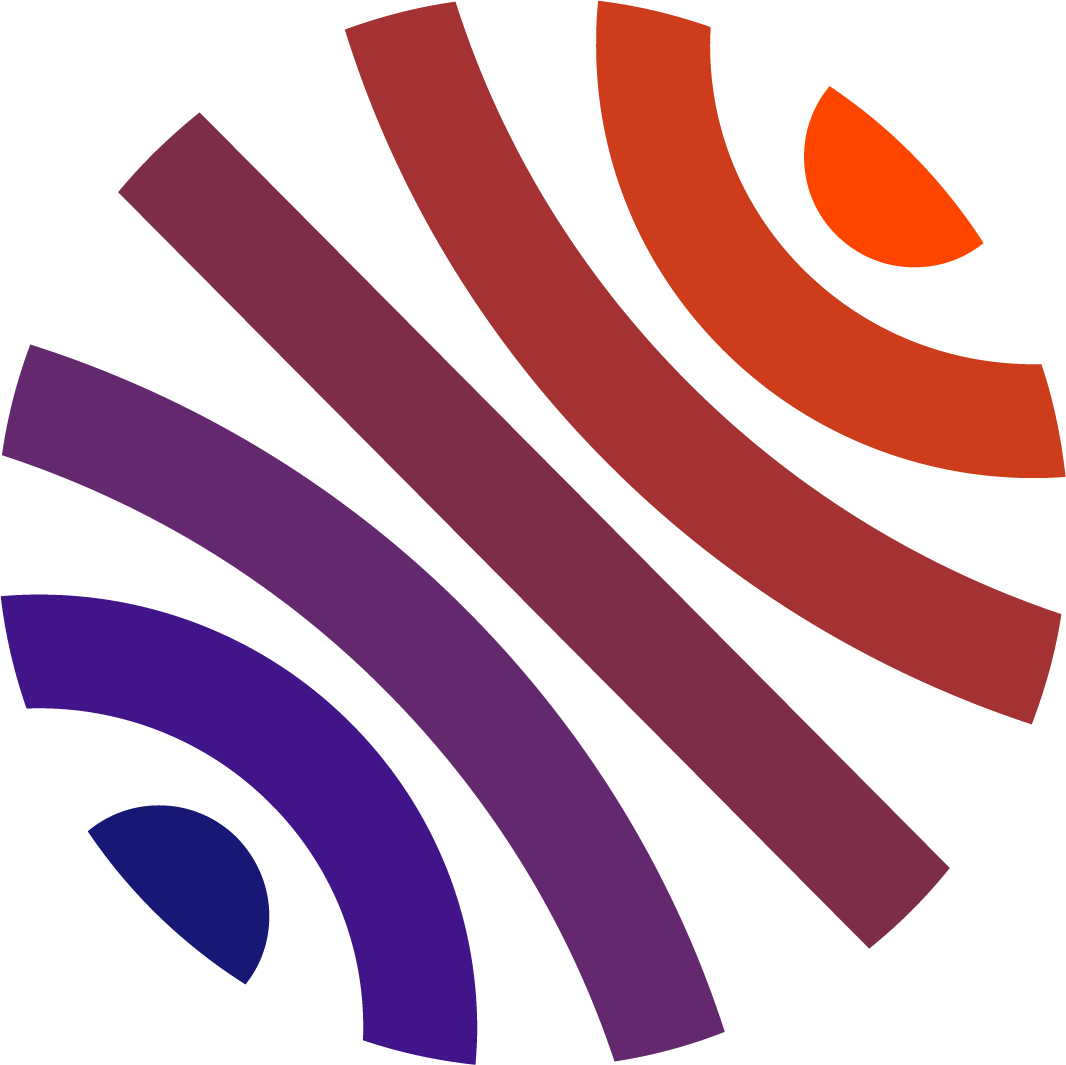
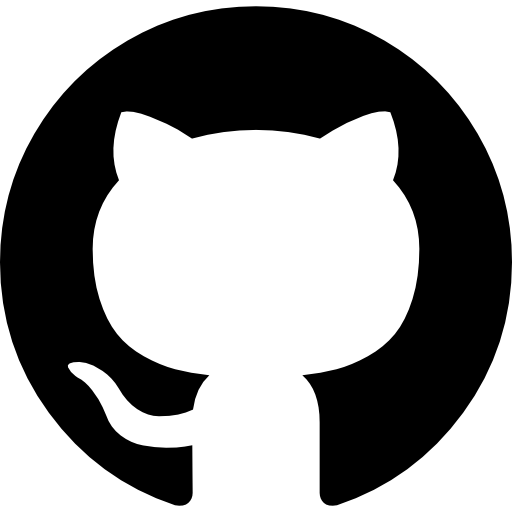
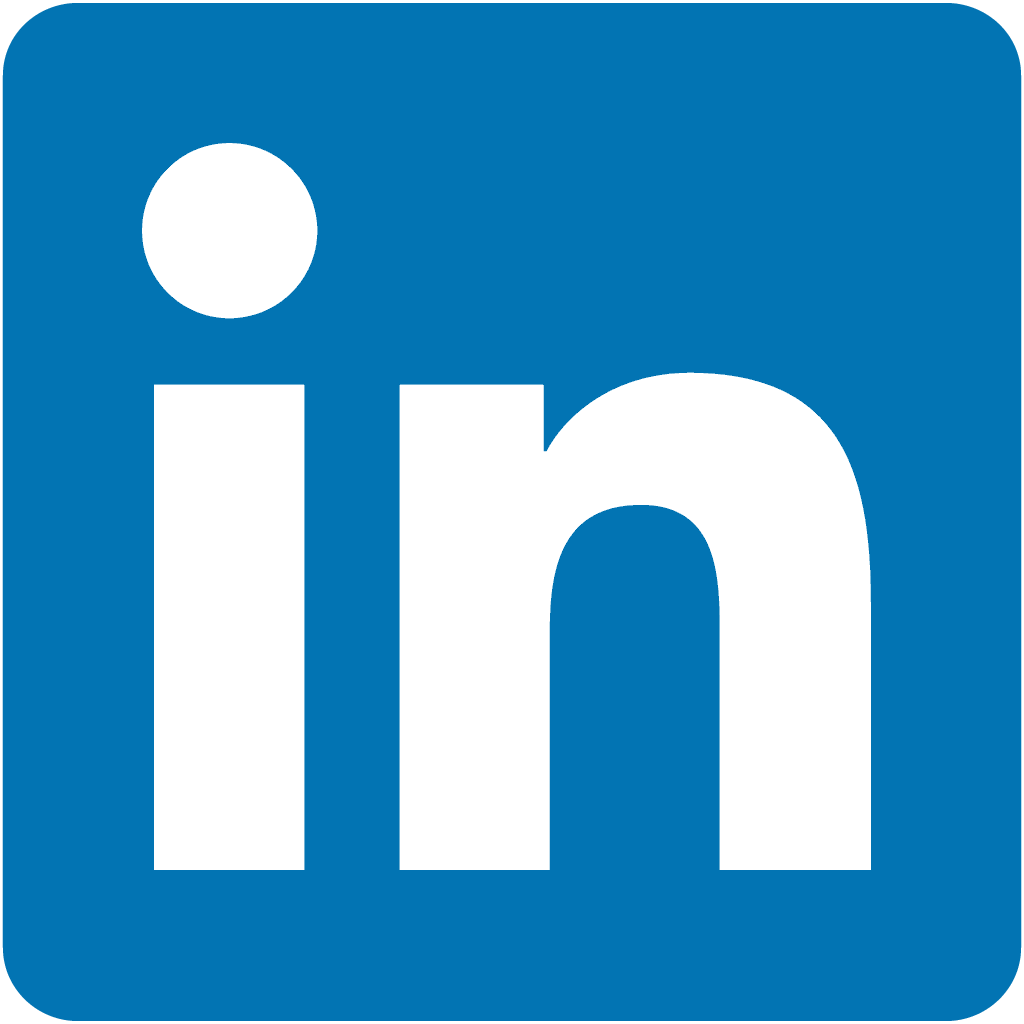
Publications list (non-exhaustive)
- Lomet, Aurore; Govaert, Gérard; Grandvalet, Yves; Model selection for Gaussian latent block clustering with the integrated classification likelihood,Advances in Data Analysis and Classification,12,,489-508,2018,Springer Berlin Heidelberg
- Kunitomo-Jacquin, Lucie; Lomet, Aurore; Daniel Geoffrey; Assessing Causal Graph Uncertainty and Optimized Multivariate Discretization Strategy,2024 International Conference on Information Processing and Management of Uncertainty in Knowledge-Based Systems (IPMU),1-8,2022
- Lomet, Aurore; Suard, Frédéric; Chèze, David; Statistical modeling for real domestic hot water consumption forecasting, Energy Procedia,70,,379-387,2015,Elsevier (https://doi.org/10.1016/j.egypro.2015.02.138)
- Kunitomo-Jacquin, Lucie; Lomet, Aurore; Poli, Jean-Philippe; Causal discovery for fuzzy rule learning,2022 IEEE International Conference on Fuzzy Systems (FUZZ-IEEE),1-8,2022,IEEE
- Arsac, Antonin; Lomet, Aurore; Poli, Jean-Philippe; Causal discovery for time series with constraint-based model and PMIME measure, Quarter of Causality conference, arXiv preprint arXiv:2305.19695,2023