The LIAD team
Inna KUCHER
Engineer-researcherInna Kucher is a research engineer specializing in machine learning applied to physics data analysis and deep learning for computer vision applications.
She completed her PhD in 2017 at the Université Paris-Saclay with the thesis work at the CEA and Cern on the subject: "Search for the Higgs boson decaying to two photons and produced in association with a pair of top quarks in the CMS experiment". The major part of the work consisted of optimizing and applying machine learning methods to analyze the data for particles and process identification. The data was produced thanks to Cern accelerators and registered with CMS detector.
Following her PhD, she joined the CMS collaboration team at Ecole Polytechnique as a postdoc in 2017. Her activities cover tools optimization for data acquisition and detector monitoring, introducing deep learning methods for particles reconstruction and identification. At the same time, she took responsibility for data collection, reconstruction, and data analysis in the working group.
Inna was recruited in 2020 as a research engineer at the Laboratory of Embedded Artificial Intelligence (DRT/LIST/DSCIN/LIAE). She worked on neural network optimization techniques, such as quantification and sparsity exploration, to produce the most efficient algorithms to be embedded in different hardware platforms (ASIC and FPGA). During 4 years in LIAE, she took responsibilities as project manager, and she conducted research in this field supervising 3 PhD students and 3 Master students.
She joined the Laboratory of Artificial Intelligence and Data Science (LIAD) in 2024. Now she focuses her research on uncertainties quantification originating from neural networks application for data analysis.
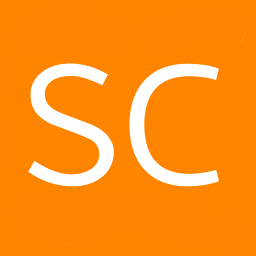
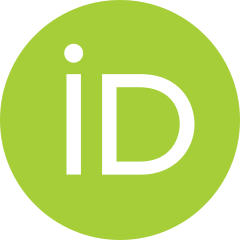
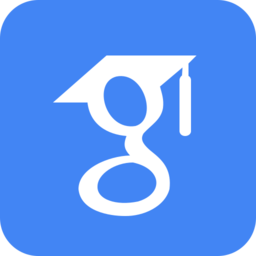
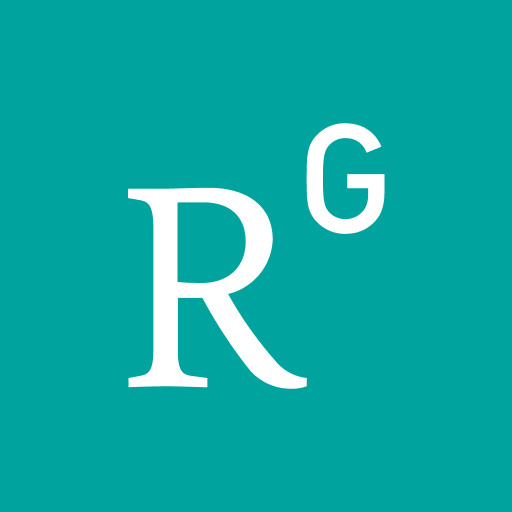
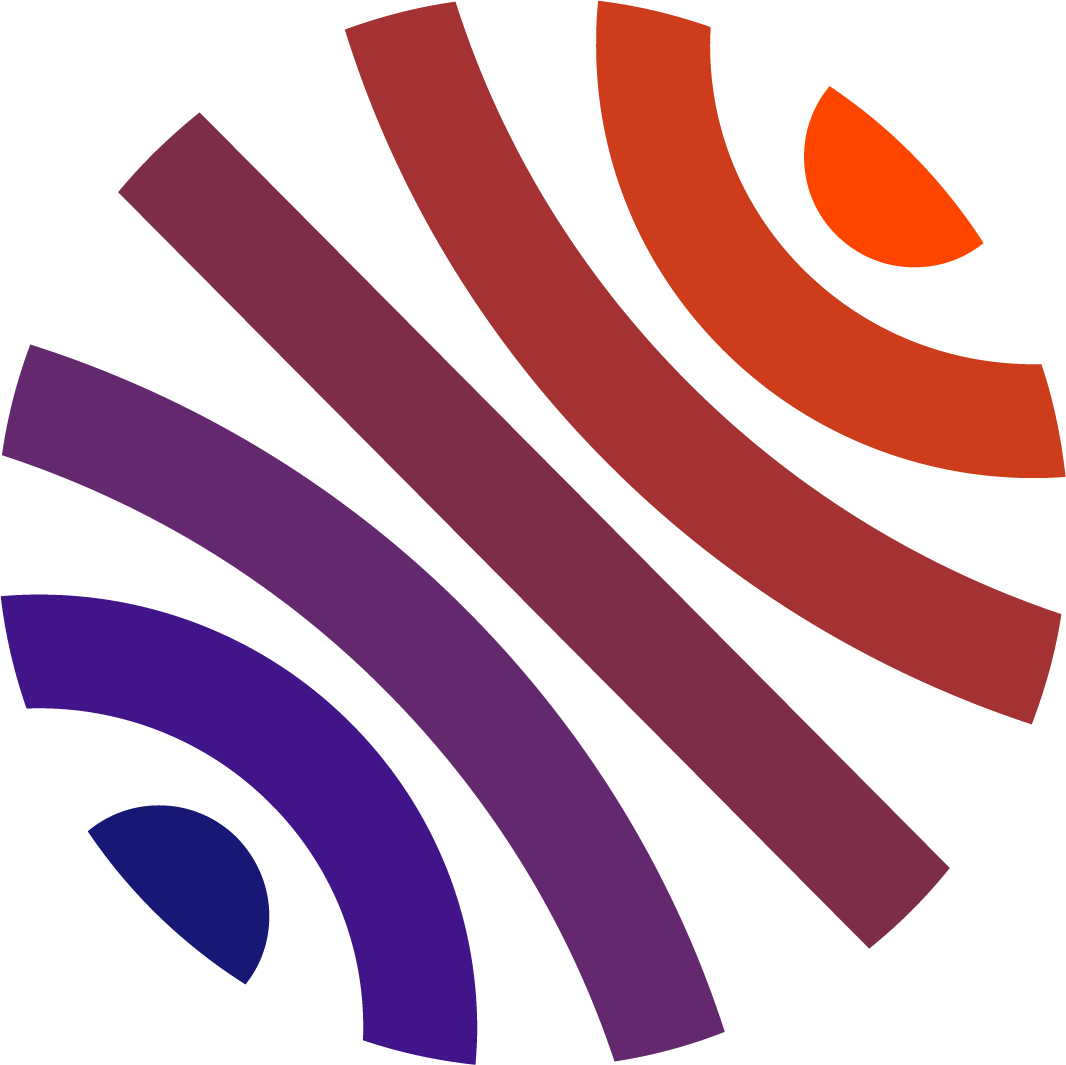
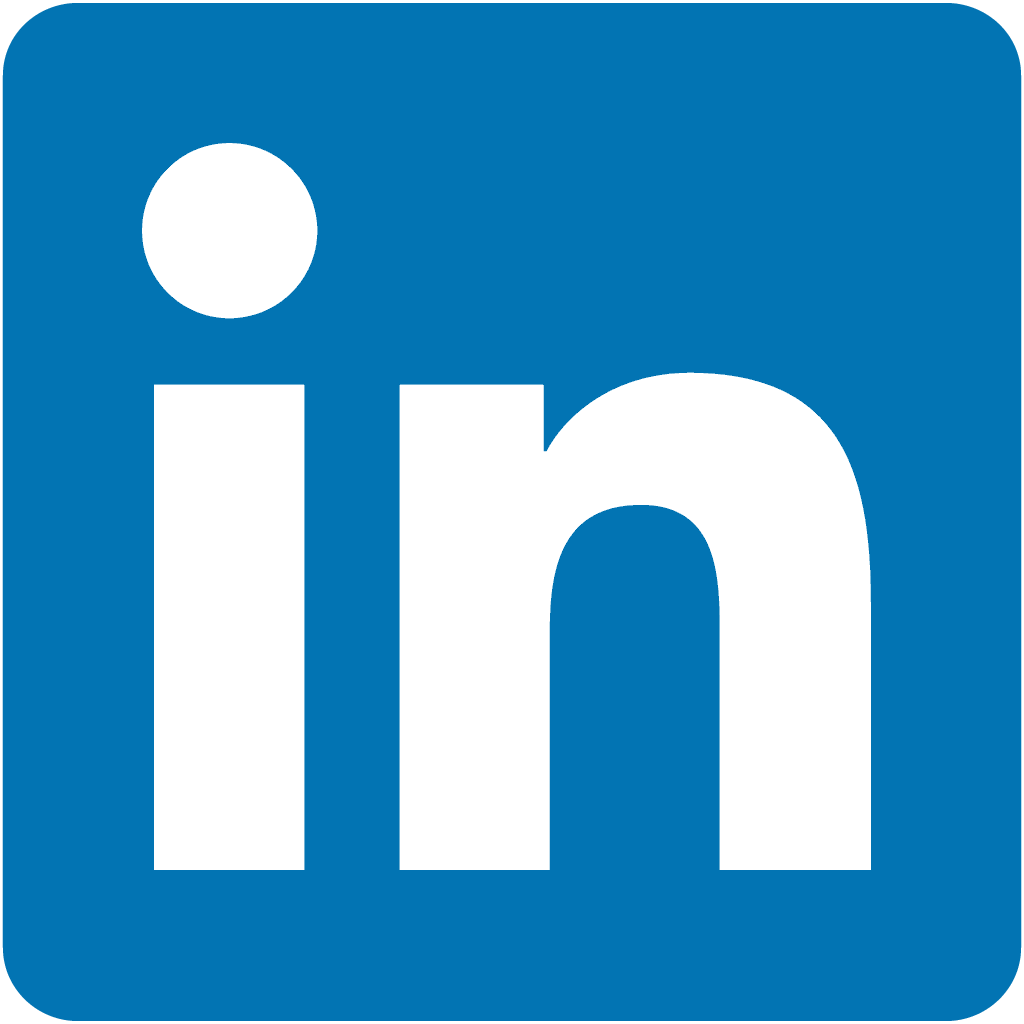
Publications list (non-exhaustive)
- I. Miro-Panades, V. Lorrain, L. Billod, I. Kucher, V. Templier, S. Choisnet, N. Ali, B. Rossigneux, O. Bichler, A. Valentian. "A 772μJ/Frame ImageNet Feature Extractor Accelerator on HD Images at 30FPS", Accepted for Lecture presentation at the 2024 IEEE Asia Pacific Conference on Circuits and Systems, to be held in Taipei, Taiwan from November 7-9, 2024
- B. Rossigneux, I. Kucher, V. Lorrain, E. Casseau. "Importance Resides In Activations: Fast Input-Based Nonlinearity Pruning", Accepted to The International Conference on Neural Information Processing (ICONIP), to be held in Auckland, New Zealand from December 2-6, 2024
- T. Pégeot, I. Kucher, A. Popescu, B. Delezoide. "Parameter-efficient Fine Tuning with Debiased Sensitivity and Constrained Low-rank Adaptation", Accepted to The International Conference on Neural Information Processing (ICONIP), to be held in Auckland, New Zealand from December 2-6, 2024
- J-L. de Melo Costa, C. Moineau , T. Allenet, I. Kucher. Centered Kernel Alignment for efficient Vision Transformer quantization, AccML and HiPEAC 2024 workshop - 6th Workshop on Accelerated Machine Learning
- S. Chali, I. Kucher, M. Duranton, J-O. Klein. Improving Normalizing Flows With the Approximate Mass for Out-of-Distribution Detection, Proceedings of the IEEE/CVF Conference on Computer Vision and Pattern Recognition (CVPR) Workshops, 2023, pp. 750-758
- B. Rossigneux, I. Kucher, V. Lorrain, E. Casseau. Surround the Nonlinearity: Inserting Foldable Convolutional Autoencoders to Reduce Activation Footprint, Proceedings of the IEEE/CVF International Conference on Computer Vision (ICCV) Workshops, 2023, pp. 1407-1411
- T. Pégeot, I. Kucher, A. Popescu, B. Delezoide. A Comprehensive Study of Transfer Learning Under Constraints, Proceedings of the IEEE/CVF International Conference on Computer Vision (ICCV) Workshops, 2023, pp. 1148-1157
- I. Miro-Panades, I. Kucher, V. Lorrain, A. Valentian. Meeting the latency and energy onstraints on timing-critical edge-AI systems, ESSCIRC 2022 EAI Workshop