The LIAD team
Riccardo FINOTELLO
Engineer-researcherRiccardo is a research engineer with a background in theoretical physics and a specialization in machine learning. His research interests span from information theory to AI for data analysis in various scientific domains.
He completed his PhD at the National Institute for Nuclear Physics (INFN) and the University of Torino with a thesis on string theory phenomenology at the interface with modern AI ("D-branes and Deep Learning: Theoretical and Computational Aspects In String Theory"). He joined CEA as a postdoc in 2021 to work on machine learning methods for Laser-Induced Breakdown Spectroscopy (LIBS) data analysis at the Laboratory of Nuclear, Isotopic and Elemental Analysis (DES/ISAS/DRMP/SPC/LANIE). In this context, he developed methods based on PCA and random matrix theory for the extraction of information from LIBS mapping experiments. He also took an interest in explainable AI (X-AI) techniques for the analysis of spectroscopy data.
Riccardo was then recruited in 2022 as a research engineer at the Laboratory of Vision for Modelisation and Localisation (DRT/LIST/DIASI/SIALV/LVML), where he continued to develop X-AI methods and techniques for data analysis and computer vision based on the use of field theory methods, such as the renormalisation group for signal detection.
He joined the Laboratory of Artificial Intelligence and Data Science (DES/ISAS/DM2S/SGLS/LIAD) in 2024, where he deals with physics and vision-inspired methodologies for data analysis, focusing on scientific and simulation data.
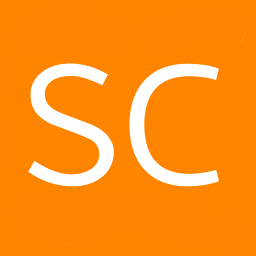
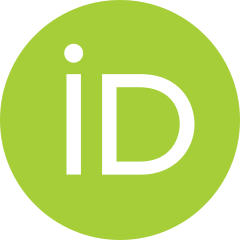
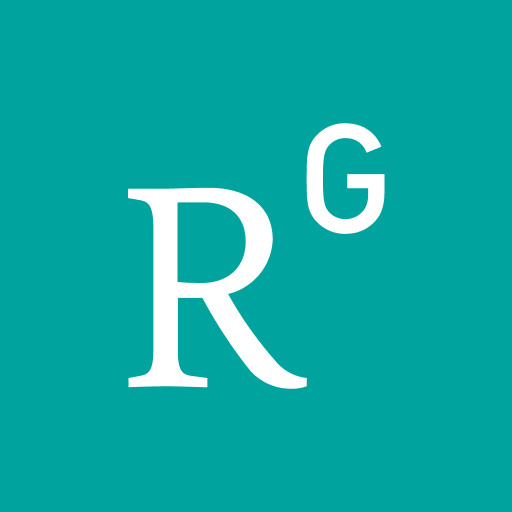
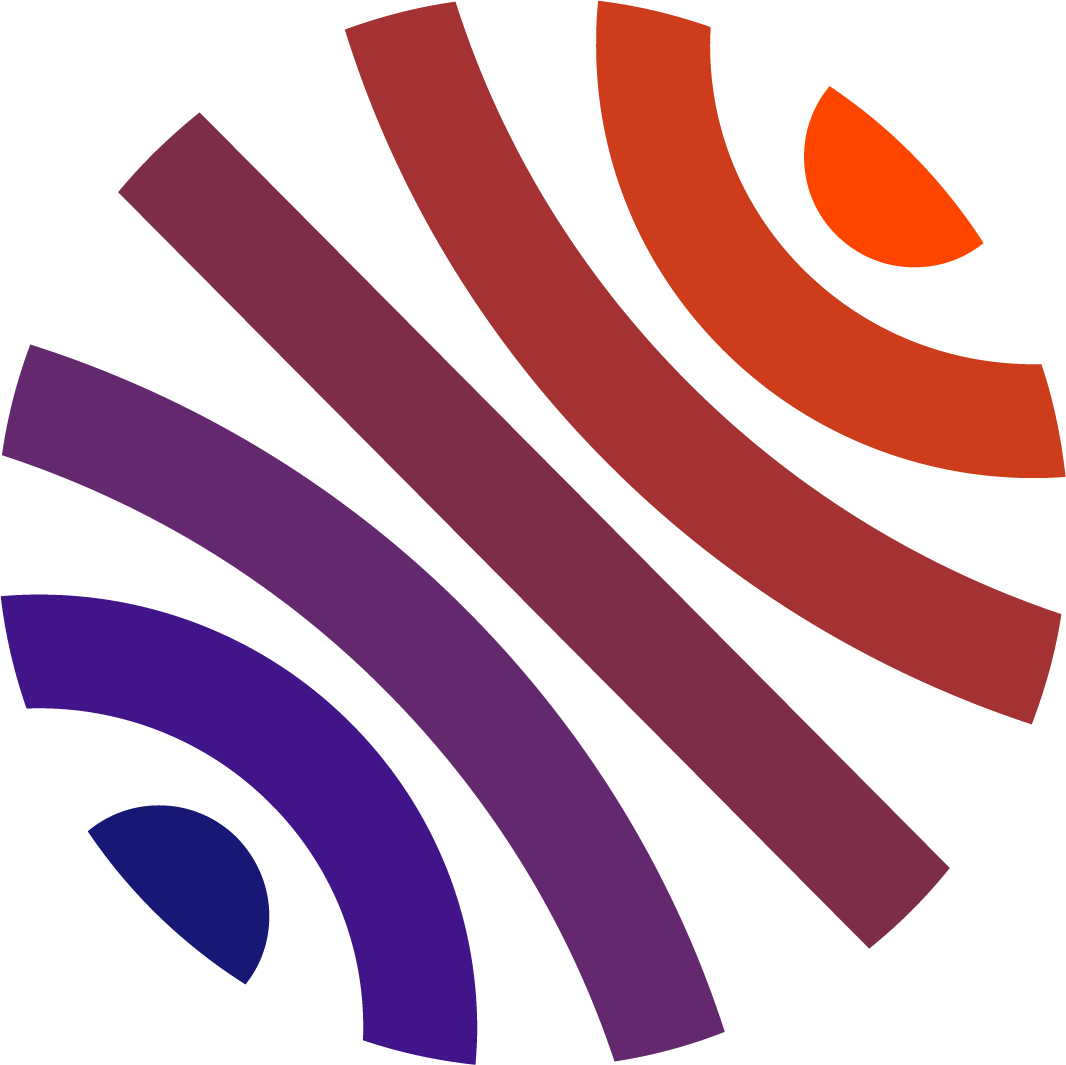
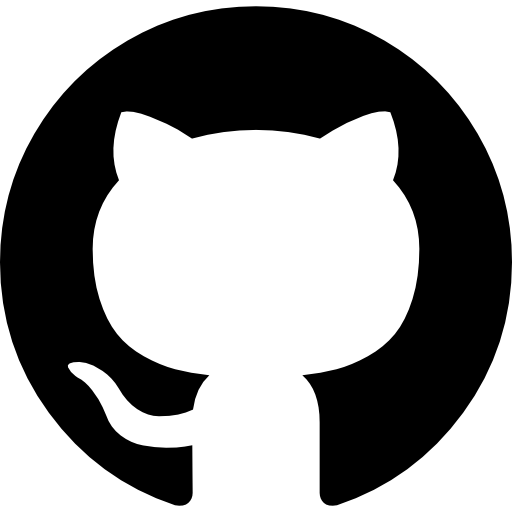
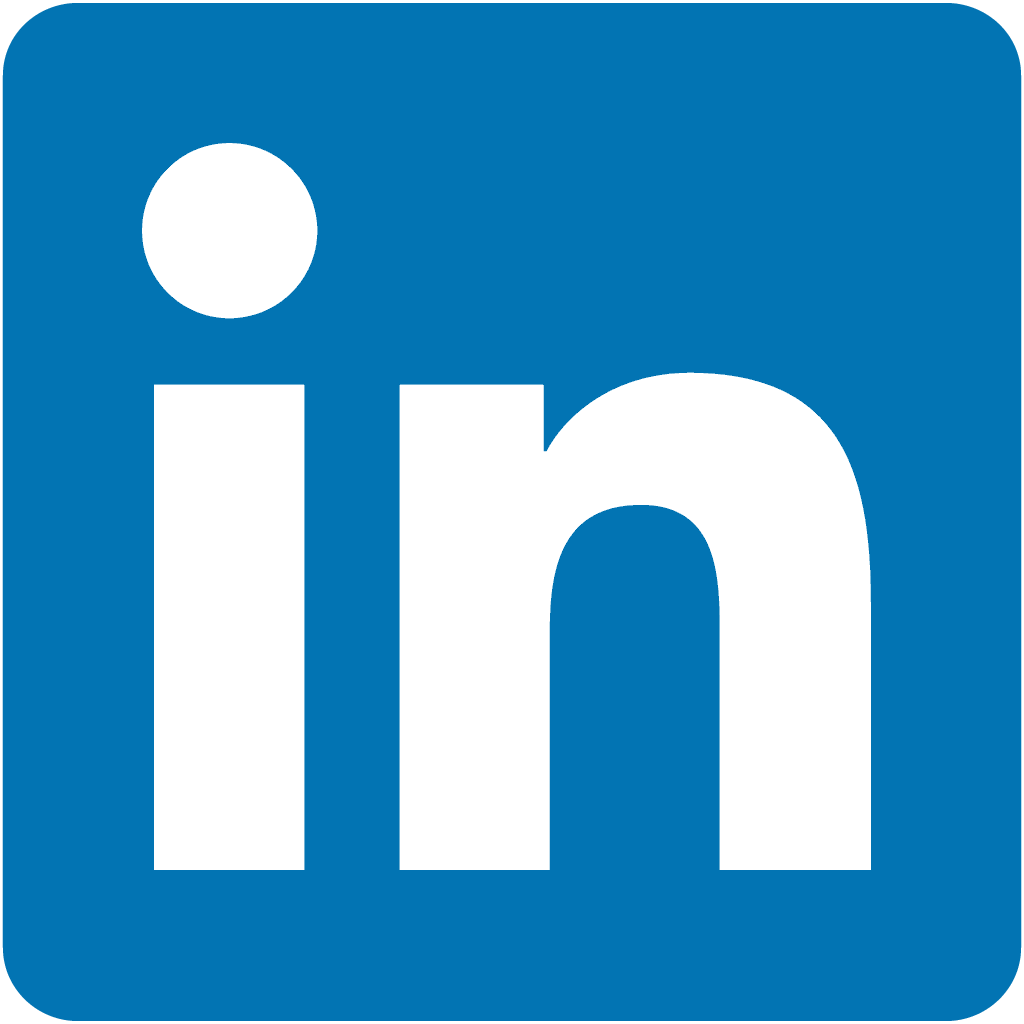
Publications list (non-exhaustive)
- Finotello, Lahoche, Ousmane Samary et al. Functional renormalization group for signal detection and stochastic ergodicity breaking. J. Stat. Mech. (2024) 083203
- Erbin, Finotello. Deep Learning Complete Intersection Calabi–Yau Manifolds, Machine Learning in Pure Mathematics and Theoretical Physics, pp. 151-181 (2023)
- Finotello et al. Trustworthiness of Laser-Induced Breakdown Spectroscopy Predictions via Simulation-based Synthetic Data Augmentation and Multitask Learning, EPJ Web Conf. 288 (2023) 01005
- Finotello et al. HyperPCA: A powerful tool to extract elemental maps from noisy data obtained in LIBS mapping of materials, Spectrochim. Acta B 192 (2022) 106418
- Erbin, Finotello. Machine learning for complete intersection Calabi-Yau manifolds: A methodological study, Phys. Rev. D 103 (2021) 126014