Documentation / Developer's manual
Available modules
Calibration,  DataServer,  Launcher,  MetaModelOptim,  Modeler,  Optimizer,  ReLauncher,  Reliability,  ReOptimizer,  Sampler,  Sensitivity,  UncertModeler,  XmlProblem,  ![]() |
Uranie / Sensitivity
v4.10.0
|
Description of the class THSIC. More...
#include <THSIC.h>
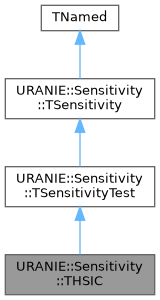
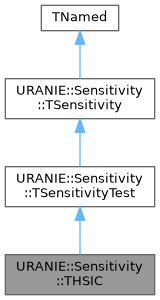
Public Types | |
enum | EEstimatedVariance { kUnknown, kStdEmpirical, kMedianeDelta } |
![]() | |
enum | ELauncher { kCode, kCodeRemote, kFunction, kRun, kUnknown } |
Public Member Functions | |
Constructors and destructor | |
THSIC () | |
Default constructor. More... | |
THSIC (URANIE::DataServer::TDataServer *tds, const char *sInputs, const char *sOutputs, Option_t *option="") | |
THSIC constructor with data contined in the TDS and the inputs and outputs attributes. More... | |
virtual | ~THSIC () |
Default destructor. More... | |
Setting and Getting attributs | |
EEstimatedVariance | getEstimatedVariance () |
Which method used to estimate the variance of the gaussian kernel ( kUnknown | kStdEmpirical | kMedianeDelta ) More... | |
void | setEstimatedVariance (EEstimatedVariance nMethod) |
Set the method used to estimate the variance of the gaussian kernel ( kUnknown | kStdEmpirical | kMedianeDelta ) More... | |
Int_t | getNPermutationSample () |
getNPermutationSample More... | |
void | setNPermutationSample (Int_t ns) |
Set the Permutation sample permutation Size (default NDefaultPermutation) More... | |
void | setThresholdPermutation (Int_t n) |
Int_t | getThresholdPermutation () |
Get the Threshold (nS/nX) to use the permutation permutation test to compute the p-value (default NDefaultThresholdPermutation) More... | |
void | setThresholdGamma (Int_t n) |
Set the Threshold (nS/nX) to use the Gamma approximation to cumpute the p-value (default NDefaultThresholdGamma) More... | |
Int_t | getThresholdGamma () |
Get the Threshold (nS/nX) to use the Gamma approximation to cumpute the p-value (default NDefaultThresholdGamma) More... | |
Parse the option | |
The options treated by the THSIC object were :
| |
virtual void | parseOption (Option_t *option="") |
Parse the option given by the user. More... | |
Printing Log | |
void | printSummary () |
Prints a concise summary of results to the terminal. More... | |
virtual void | printLog (Option_t *option="") |
Prints all attributes of the class for the current instance. More... | |
All methods used for elementary tasks | |
void | generateHMatrix (Option_t *option="") |
generate the \( H \) matrix More... | |
TMatrixD | doubleCenteringRect (const TMatrixD &matK) |
Centers the rows and columns of the provided matrix. More... | |
TMatrixDSym | doubleCentering (const TMatrixDSym &matK) |
Centers the rows and columns of the provided matrix. More... | |
Double_t | estimateSigma (const char *sAtt, Option_t *option="") |
Computes the bandwidth parameter of the specified attribute. More... | |
TMatrixDSym | getMatrixKGaussian (const char *sAtt, Double_t dTheta) |
Constructs the Gram matrix associated with the specified attribute. More... | |
Double_t | biasedHSIC (const TMatrixDSym &matKx, const TMatrixDSym &matKy) |
Computes the V-statistic estimate of \( HSIC(X, Y) \) where \( X \). The computation is based on the Gram matrices \( K_{x} \) and \( K_{y} \) which are constructed beforehand from the observations of \( X \) and \( Y \). More... | |
Double_t | unbiasedHSIC (const TMatrixDSym &matKx, const TMatrixDSym &matKy) |
Computes the U-statistic estimate of \( HSIC(X, Y) \). The computation is based on the Gram matrices \( K_{x} \) and \( K_{y} \) which are constructed beforehand from the observations of \( X \) and \( Y \). More... | |
Double_t | getProductOfNorms (const TMatrixDSym &matKx, const TMatrixDSym &matKy) |
Estimates the product of the squared Hilbert-Schmidt (HS) norms of the cross-covariance operators \( C_{xx} \) and \( C_{yy} \). More... | |
void | permuteKMatrix (const TMatrixDSym &theMatK, TList *tlstPerm, TList *tlstPermMat) |
Given a Gram matrix and a list of permutations, constructs the permuted matrices and store them in a list. More... | |
Overloaded methods | |
virtual void | generateSample (Option_t *option="") |
No action is performed, but a warning is returned. More... | |
void | computeIndexes (Option_t *option="") |
Calls the method evaluateIndexes. More... | |
void | evaluateIndexes (Option_t *option) |
Applies the HSIC methodology. More... | |
void | drawIndexes (TString sTitle, const char *select="", Option_t *option="") |
Draw the indexes computed by the method. More... | |
![]() | |
TSensitivityTest () | |
Default constructor. More... | |
TSensitivityTest (URANIE::DataServer::TDataServer *tds, const char *varexpinput, const char *varexpoutput, Option_t *option="") | |
Default constructor. More... | |
virtual | ~TSensitivityTest () |
Default destructor. More... | |
![]() | |
TSensitivity () | |
Default constructor. More... | |
TSensitivity (URANIE::DataServer::TDataServer *tds, const char *fcn, Int_t ns, const char *varexpinput="", const char *varexpoutput="", Option_t *option="") | |
Default constructor with the name of a function. More... | |
TSensitivity (URANIE::DataServer::TDataServer *tds, void(*fcn)(Double_t *, Double_t *), const char *varexpinput, const char *varexpoutput, Int_t ns, Option_t *option="") | |
TSensitivity (URANIE::DataServer::TDataServer *tds, const char *varexpinput, const char *varexpoutput, Option_t *option="") | |
Default constructor. More... | |
TSensitivity (URANIE::DataServer::TDataServer *tds, URANIE::Launcher::TCode *code, Int_t ns, Option_t *option="") | |
Default constructor with TCode arg. More... | |
TSensitivity (URANIE::DataServer::TDataServer *tds, URANIE::Relauncher::TRun *run, Int_t ns, Option_t *option="") | |
Default constructor with TRun arg. More... | |
virtual | ~TSensitivity () |
Default destructor. More... | |
Int_t | getID () |
virtual TTree * | getResultTuple (bool commonresulttuple=true) |
Get the result ntuple (default parameter unused but for Morris method) More... | |
double | getValue (const char *sorder="", const char *sinputname="", const char *sselect="") |
vector< int > * | getAttributeElements (string str) |
void | setFunction (void(*fct)(Double_t *, Double_t *), Int_t nx=-1, Int_t ny=1) |
TString | getFunctionName () |
void | setSeed (UInt_t nval) |
UInt_t | getSeed () |
virtual void | setMethodName (const char *str) |
Set the Method name. More... | |
const char * | getMethodName () |
Get the method name. More... | |
Bool_t | getNoIntermediateSaved () |
Get the noIntermediateSaved flag. More... | |
const char * | getIteratorName () |
Get the name of the iterator attribut of the method. More... | |
void | setSensitivityIteratorName (const char *str) |
Set the iterator name devoted to compute sensitivity indexes. More... | |
void | setTimeName (TString sname) |
Set the name of the time attribute (only one) More... | |
TString | getTimeName () |
Get the name of the time attribute. More... | |
virtual void | setDrawProgressBar (Bool_t bbool=kTRUE) |
Set the "draw progress bar" flag. More... | |
void | setUsingErrors (bool thebool) |
Set the "using error results anyway" option. More... | |
Bool_t | getDrawProgressBar () |
Get the clean flag. More... | |
Bool_t | isInputCorrelated () |
TMatrixD | getMatrixInputCorrelation () |
Int_t | getNInput () |
Get the number of input attributes. More... | |
Int_t | getNOutput () |
Get the number of output attributes. More... | |
void | setInputCorrelationMatrix (TMatrixD Corr) |
void | checkOutputRequested (string attlist, bool fromoption=false) |
Check the output list requested by the user. More... | |
void | computeIndexes (Option_t *option="") |
Compute the Sensitivity Indexes. More... | |
void | fillIndex (const char *sinputname, const char *sorder, Double_t dval, const char *algo="", Double_t dvalCILower=-1.0, Double_t dvalCIUpper=-1.0) |
Method to fill in the tree the value of Sensitivity indexes for an input attribute. More... | |
virtual void | createTuple (Option_t *option="") |
virtual void | setLog () |
void | unsetLog () |
void | changeLog () |
Bool_t | getLog () |
void | setNLauncher (ELauncher codeLauncher) |
Static Public Member Functions | |
Static methods | |
static TString | convertRule (EEstimatedVariance evRule) |
Outputs the string representation of any enumerator of EEstimatedVariance. More... | |
Private Attributes | |
Private and Public attributes | |
EEstimatedVariance | _nEstimatedVariance |
< The H matrix defined by H := ( Kronecker(i,j) - 1/nS) More... | |
Int_t | _nPermutationSample |
the method to compute the variance for the gaussian kernel More... | |
Int_t | _nThresholdPermutation |
The number of permutation sample ( permutation ) (default NDefaultPermutation) More... | |
Int_t | _nThresholdGamma |
The Threshold (nS/nX) to use the permutation permutation test to cumpute the p-value (default 5) More... | |
Additional Inherited Members | |
![]() | |
URANIE::DataServer::TDataServer * | _tds |
Pointeur vers un TDS. More... | |
TList * | _listOfInputAttributes |
List of the input branches. More... | |
TList * | _listOfOutputAttributes |
List of the input branches. More... | |
TString | _sTimeAttribute |
The name of the Time attribute. More... | |
Int_t | _nS |
The number of simulation or other information depend on the used method. More... | |
Int_t | _nX |
Dimension of the input. More... | |
UInt_t | _nY |
Dimension of the target. More... | |
UInt_t | _nElY |
Number of element for one selected output. More... | |
Int_t | _nbOut |
Total number of Output to be considered. More... | |
Int_t | _iOut |
counter for output More... | |
unsigned int | _iy |
iterator over number of element More... | |
unsigned int | _iely |
iterator over number of element More... | |
ELauncher | _nLauncher |
The type of launcher. More... | |
TString | _sFunctionName |
The Name of the evaluatuor. More... | |
URANIE::Launcher::TCode * | _code |
The tcode. More... | |
URANIE::Relauncher::TRun * | _run |
TObjArray * | _drawingGarbageCollector |
Garbage collector for prints. More... | |
Int_t | _nSeed |
The seed of the random generator. More... | |
Bool_t | _bChosenOutputs |
Fact that the input list is provided or not. More... | |
Bool_t | _blog |
Boolean for edit the log. More... | |
Bool_t | _bquiet |
Quiet mode. More... | |
Bool_t | _bdrawProgressBar |
Boolean to know if the progress bar has to be drawn. More... | |
Bool_t | _bnoIntermediateSaved |
Boolean to know if the progress bar has to be drawn. More... | |
TString | _sIteratorName |
The specific iterator attribute for the method. More... | |
TString | _sMethodName |
The method name. More... | |
TString | _sSelectedOutput |
The output. More... | |
TString | _sSelectedInput |
The input. More... | |
map< string, unsigned int > | _mAttributeSize |
Map of size of element for attribute;. More... | |
map< string, vector< int > > | _mAttributeElements |
Map of Elements number to run (if vector subselection is requested) More... | |
vector< string > | _vOutputNames |
Name of the output. More... | |
TCanvas * | _canvas |
Canvas object to deal with. More... | |
void(* | _pFunction )(double *, double *) |
TTree * | _ntresult |
The TTree of results. More... | |
![]() | |
virtual void | preTreatment () |
PreTreatment for every output. More... | |
virtual void | postTreatment () |
PostTreatment for every output. More... | |
void | setNoIntermediateSaved (Bool_t bbool=kTRUE) |
Set the "only final file" flag. More... | |
void | checkCanvasCreation (bool newcan) |
Create a canvas if needed. More... | |
void | drawIndexesHistogram (TString sTitre, const char *select="", Option_t *option="") |
Draws indexes with an histogram. More... | |
void | drawIndexesPie (TString sTitre, const char *select="", Option_t *option="") |
Draws indexes with an pie chart. More... | |
![]() | |
Char_t | _sOutputAttribute [MAXLENGTHSTRING] |
The name of the output attribute. More... | |
Char_t | _sInputAttribute [MAXLENGTHSTRING] |
The name of the input attribute. More... | |
Char_t | _sOrder [MAXLENGTHSTRING] |
The order of sensitivity indexes. More... | |
Char_t | _sMethod [MAXLENGTHSTRING] |
The name of the method. More... | |
Char_t | _sAlgorithm [MAXLENGTHSTRING] |
The name of the algorithm to compute the index. More... | |
Double_t | _valSobolCrt |
The value of sensitivity indexes. More... | |
Double_t | _valSobolCILower |
The value of lower Condidence Interval (95) More... | |
Double_t | _valSobolCIUpper |
The value of upper Condidence Interval (95) More... | |
TMatrixD | _minputCorr |
Input correlation matrix if sample needs to be correlated. More... | |
Bool_t | _bisInputCorrelated |
State whether the input correlation matrix is set. More... | |
Bool_t | _bgoingThroughError |
State whether the error must not block the computation. More... | |
Detailed Description
Description of the class THSIC.
Description of the class THSIC.
Introduction
The goal of this class is to find the list of input attributes which are dependent with the output attributes using the "HSIC" values (for "Hilbert-Schmidt Independence Criterion"). This approach was introduced by Gretton (2005, 2007).
Moreover, in the same time, we compute the \( R^{2}_{HSIC} \) proposed by Da Veiga (2015) given by the formula
\[ R^{2}_{HSIC} ( X, Y) \; = \; \frac{ HSIC ( X, Y) }{\sqrt{ HSIC ( X, X) \, \times \, HSIC ( Y, Y)}} \]
Draw Indexes
We use a "Pareto chart" to draw the "p-Values" and the \( R^{2}_{HSIC} \).
Bibliography
- Da Veiga, S. " Global Sensitivity Analysis with Dependence Measures ", https://arxiv.org/abs/1311.2483, 2013
- Zhang, Q., Filippi, S., Gretton, A., Sejdinovic, D., "Large-Scale Kernel Methods for Independence Testing", 2016
- Gretton, A., Fukumizu, K., Teo, C.H., Song, L., Schoelkopf, B., et Smola, A., "A Kernel Statistical Test of Independence,", NIPS 2007
- Gretton, A., Herbrich, R., Smola, A., Bousquet, O., et Schoelkopf, B., "Kernel Methods for Measuring Independence", Journal of Machine Learning Research, 6 , pp.2075–2129, 2005
- The Pareto chart, https://en.wikipedia.org/wiki/Pareto_chart
Member Enumeration Documentation
◆ EEstimatedVariance
Constructor & Destructor Documentation
◆ THSIC() [1/2]
URANIE::Sensitivity::THSIC::THSIC | ( | ) |
Default constructor.
Referenced by ClassImp().
◆ THSIC() [2/2]
URANIE::Sensitivity::THSIC::THSIC | ( | URANIE::DataServer::TDataServer * | tds, |
const char * | sInputs, | ||
const char * | sOutputs, | ||
Option_t * | option = "" |
||
) |
THSIC constructor with data contined in the TDS and the inputs and outputs attributes.
- Parameters
-
tds sInputs sOutputs option (Option_t*) option to pass [""]
◆ ~THSIC()
|
virtual |
Default destructor.
Referenced by ClassImp().
Member Function Documentation
◆ biasedHSIC()
Double_t URANIE::Sensitivity::THSIC::biasedHSIC | ( | const TMatrixDSym & | matKx, |
const TMatrixDSym & | matKy | ||
) |
Computes the V-statistic estimate of \( HSIC(X, Y) \) where \( X \). The computation is based on the Gram matrices \( K_{x} \) and \( K_{y} \) which are constructed beforehand from the observations of \( X \) and \( Y \).
The formula of the V-statistic estimator can be found in: [Zhang et al., 2018] "Large-scale kernel methods for independence testing", Statistics and Computing", page 117, Eq (9).
Let \( \widetilde{K}_{x} \) be the double-centered Gram matrix built from the observations of \( X \). Let \( \widetilde{K}_{y} \) be the double-centered Gram matrix built from the observations of \( Y \).
The formula of the V-statistic estimator is given by:
\[ \widehat{HSIC}_{V}(X, Y) \, = \, \frac{1}{nS^2} \, Tr( \widetilde{K}_{x} \widetilde{K}_{y} ) \]
- Parameters
-
matKx (TMatrixDSym) The Gram matrix built from the observations of \( X \). matKy (TMatrixDSym) The Gram matrix built from the observations of \( Y \).
- Returns
- (Double_t) The value of the V-statistic estimate of \( HSIC(X, Y) \).
Referenced by ClassImp().
◆ computeIndexes()
void URANIE::Sensitivity::THSIC::computeIndexes | ( | Option_t * | option = "" | ) |
Calls the method evaluateIndexes.
- Parameters
-
option (Option_t*) Specifies the option to pass to the method evaluateIndexes.
Referenced by ClassImp().
◆ convertRule()
|
static |
Outputs the string representation of any enumerator of EEstimatedVariance.
- Parameters
-
evRule (EEstimatedVariance) enumerator of EEstimatedVariance that must be represented by a string
Referenced by ClassImp().
◆ doubleCentering()
TMatrixDSym URANIE::Sensitivity::THSIC::doubleCentering | ( | const TMatrixDSym & | matK | ) |
Centers the rows and columns of the provided matrix.
- Parameters
-
matK (TMatrixDSym) The \( n \)-by- \( n \) matrix \( K \) that needs to be centered.
- Returns
- (TMatrixDSym) The double-centered matrix produced from $ \( K \).
It is defined as \( \widetilde{K} := H \, K \, H \) where: \( H \) is the centering matrix of dimension \( n \).
Note that the sum of each row (resp. column) of \( \widetilde{K} \) is equal to zero.
Referenced by ClassImp().
◆ doubleCenteringRect()
TMatrixD URANIE::Sensitivity::THSIC::doubleCenteringRect | ( | const TMatrixD & | matK | ) |
Centers the rows and columns of the provided matrix.
- Parameters
-
matK (TMatrixD) The \( n \)-by- \( m \) matrix \( K \) that needs to be centered.
- Returns
- (TMatrixD) The double-centered matrix produced from $ \( K \).
It is defined as \( \widetilde{K} := H_n \, K \, H_m \) where: \( \forall \, r \geq 1 \), \( H_r \) is the centering matrix of dimension \( r \).
Note that the sum of each row (resp. column) of \( \widetilde{K} \) is equal to zero.
Referenced by ClassImp().
◆ drawIndexes()
|
virtual |
Draw the indexes computed by the method.
- Parameters
-
sTitle (TString) the title of the graph select (const char*) the select argument option (Option_t*) option to pass [""]
Reimplemented from URANIE::Sensitivity::TSensitivity.
Referenced by ClassImp().
◆ estimateSigma()
Double_t URANIE::Sensitivity::THSIC::estimateSigma | ( | const char * | sAtt, |
Option_t * | option = "" |
||
) |
Computes the bandwidth parameter of the specified attribute.
This function calculates the bandwidth parameter based on the observations of the specified attribute, which are stored in the attached TDataServer.
- Parameters
-
sAtt (const char*) The name of the attribute for which the bandwith parameter is to be computed. option (Option_t*) The rule of thumb used to compute the bandwidth parameter from the data. The user can choose between: - "empiri": empirical standard deviation,
- "median": median of pair distances.
- Returns
- (Double_t) The computed value of the bandwidth parameter.
Referenced by ClassImp().
◆ evaluateIndexes()
|
virtual |
Applies the HSIC methodology.
For each input-output pair \( (X_i, Y_j) \), this function estimates three sensitivity indices:
- the (standard) HSIC index,
- the normalized HSIC index (also called R2-HSIC index),
- the p-value of the independence test.
- Parameters
-
option (Option_t*) Some possible options (level of verbosity, estimator type, test procedure).
By default:
- The function operates in verbose mode, providing many details.
- HSIC indices (and R2-HSIC indices) are estimated with V-statistics, which yields (slightly) biased but non-negative estimates.
- The p-values are estimated with the asymptotic test procedure (based on asymptotic formulas).
The option
argument allows customization. It should be a concatenation of substrings, such as: "[option_1]" or "[option_1],[option_2]" or "[option_1],[option_2],[option_3]".
Valid substrings include:
- "quiet" to enable the silent mode (instead of the verbose mode),
- "unbiased" to estimate HSIC indices with U-statistics (instead of V-statistics),
- "nperm=500" to estimate the p-values with the permutation-based test procedure (instead of the asymptotic test procedure). This procedure will apply \( B = 500 \) random permutations on the output data before estimating the p-value. Note that the number of permutations can be set to any integer greater than \( 7 \).
Implements URANIE::Sensitivity::TSensitivity.
Referenced by ClassImp().
◆ generateHMatrix()
void URANIE::Sensitivity::THSIC::generateHMatrix | ( | Option_t * | option = "" | ) |
generate the \( H \) matrix
The \( H \) matrix is defined by the formula
\[ H (i, j) \, = \, 1\!\!I_{nS, nS} \: - \: \frac{1}{nS} \: \delta_{i=j} \]
- Parameters
-
option (Option_t *) option to pass [""]
Referenced by ClassImp().
◆ generateSample()
|
virtual |
No action is performed, but a warning is returned.
Remember that any THSIC instance is created from an already filled TDataServer.
- Parameters
-
option (Option_t*) Useless here.
Implements URANIE::Sensitivity::TSensitivity.
Referenced by ClassImp().
◆ getEstimatedVariance()
|
inline |
Which method used to estimate the variance of the gaussian kernel ( kUnknown | kStdEmpirical | kMedianeDelta )
- kStdEmpirical : the \( \sigma \) parameters is estimated by the emperical standatd deviation of the attributes
- kMedianeDelta : use the median distance between points
- Returns
- the method used to estimated the variance of the gaussian kernel ( kStdEmpirical | kMedianeDelta )
References _nEstimatedVariance.
◆ getMatrixKGaussian()
TMatrixDSym URANIE::Sensitivity::THSIC::getMatrixKGaussian | ( | const char * | sAtt, |
Double_t | dTheta | ||
) |
Constructs the Gram matrix associated with the specified attribute.
This function uses the observations of the specified attribute, stored in the attached TDataServer, for kernelization. The kernel function applied to the data is a Gaussian kernel, whose parameter is provided as second argument.
- Parameters
-
sAtt (const char*) The name of the attribute for which the Gram matrix is to be constructed. dTheta (Double_t) The parameter of the Gaussian kernel.
- Returns
- (TMatrixDSym) The constructed Gram matrix.
Referenced by ClassImp().
◆ getNPermutationSample()
|
inline |
getNPermutationSample
- Returns
- The number of Permutation sample (permutation zize) to estimate the pValues
References _nPermutationSample.
◆ getProductOfNorms()
Double_t URANIE::Sensitivity::THSIC::getProductOfNorms | ( | const TMatrixDSym & | matKx, |
const TMatrixDSym & | matKy | ||
) |
Estimates the product of the squared Hilbert-Schmidt (HS) norms of the cross-covariance operators \( C_{xx} \) and \( C_{yy} \).
This quantity appears in the asymptotic variance of the test statistic (either the U-statistic or V-statistic estimator of the HSIC) under the null hypothesis. The estimator is equal to the mean of all the off-diagonal coefficients of the following matrix: \( \left( \widetilde{K}_{x} \odot \widetilde{K}_{y} \right)^{ \odot 2} \) where:
- \( \widetilde{K}_{x} \) and \( \widetilde{K}_{y} \) are the double-centered matrices derived from \( K_{x} \) and \( K_{y} \),
- \( \odot \) denotes the Hadamard product (element-wise product).
Further details can be found in [Gretton et al., 2007]. Note that the product of the squared HS norms appears in Theorem 4 and Appendix A.2.
- Parameters
-
matKx (TMatrixDSym) The input Gram matrix \( K_{x} \). matKy (TMatrixDSym) The output Gram matrix \( K_{y} \).
- Returns
- (Double_t) An estimate of the product of the two squared HS norms.
Referenced by ClassImp().
◆ getThresholdGamma()
|
inline |
Get the Threshold (nS/nX) to use the Gamma approximation to cumpute the p-value (default NDefaultThresholdGamma)
- Returns
- The current Threshold (nS/nX) for using the Gamma approximation
References _nThresholdGamma.
◆ getThresholdPermutation()
|
inline |
Get the Threshold (nS/nX) to use the permutation permutation test to compute the p-value (default NDefaultThresholdPermutation)
- Returns
- The current Threshold (nS/nX) for using the permutation permutation test
References _nThresholdPermutation.
◆ parseOption()
|
virtual |
Parse the option given by the user.
- Parameters
-
option (Option_t) the string of option
Reimplemented from URANIE::Sensitivity::TSensitivity.
Referenced by ClassImp().
◆ permuteKMatrix()
void URANIE::Sensitivity::THSIC::permuteKMatrix | ( | const TMatrixDSym & | theMatK, |
TList * | tlstPerm, | ||
TList * | tlstPermMat | ||
) |
Given a Gram matrix and a list of permutations, constructs the permuted matrices and store them in a list.
Let us recall that a permutation is a one-to-one map \( \sigma: \{ 1, \ldots, nS \} \rightarrow \{ 1, \ldots, nS \} \). For a given Gram matrix \( K = \left[ K(i,j) \right]_{1 \leq i, j \leq nS} \) and a given permutation \( \sigma \), the permuted Gram matrix is defined as:
\[ K_{\sigma} := \left[ K \left( \sigma(i), \sigma(j) \right) \right]_{1 \leq i, j \leq nS} \]
.
- Parameters
-
theMatK (TMatrixDSym) Gram matrix that is to be permuted. tlstPerm (TList*) Pointer to the list of permutations. Each element in *tlstPerm is a pointer to a TPatternsEventList (class defined in the DataServer module). The first element in *tlstPerm must be a pointer to a TPatternsEventList representing the identity matrix. tlstPermMat (TList*) Pointer to the list of permuted Gram matrices. An empty list must be passed as argument. For each new permutation found in *tlstPerm, theMatK is permuted accordingly, and a pointer to the resulting TMatrixDSym is stored in *tlstKPermMat.
Referenced by ClassImp().
◆ printLog()
|
virtual |
Prints all attributes of the class for the current instance.
- Parameters
-
option (Option_t*) Directly forwarded to the TSensitivityTest::printLog method.
Reimplemented from URANIE::Sensitivity::TSensitivityTest.
Referenced by ClassImp().
◆ printSummary()
void URANIE::Sensitivity::THSIC::printSummary | ( | ) |
Prints a concise summary of results to the terminal.
Specifically, for each input-output pair \( (X_i, Y_j) \), the following results are displayed:
- the HSIC index,
- the normalized HSIC index (or R2-HSIC index),
- the p-value of the independence test.
Referenced by ClassImp().
◆ setEstimatedVariance()
void URANIE::Sensitivity::THSIC::setEstimatedVariance | ( | EEstimatedVariance | nMethod | ) |
Set the method used to estimate the variance of the gaussian kernel ( kUnknown | kStdEmpirical | kMedianeDelta )
- Parameters
-
nMethod (EEstimatedVariance) One of the ( kStdEmpirical | kMedianeDelta ) choice
- Exceptions
-
UErrorExceptions for the case (kUnknown)
Referenced by ClassImp().
◆ setNPermutationSample()
void URANIE::Sensitivity::THSIC::setNPermutationSample | ( | Int_t | ns | ) |
Set the Permutation sample permutation Size (default NDefaultPermutation)
- Parameters
-
ns size of the permutation permutation size
Referenced by ClassImp().
◆ setThresholdGamma()
void URANIE::Sensitivity::THSIC::setThresholdGamma | ( | Int_t | n | ) |
Set the Threshold (nS/nX) to use the Gamma approximation to cumpute the p-value (default NDefaultThresholdGamma)
- Parameters
-
n (Int_t) the Threshold (nS/nX) for using the Gamma approximation
Referenced by ClassImp().
◆ setThresholdPermutation()
void URANIE::Sensitivity::THSIC::setThresholdPermutation | ( | Int_t | n | ) |
Set the threshold (nS/nX) to use the permutation permutation test to cumpute the p-value (default NDefaultThresholdPermutation)
Referenced by ClassImp().
◆ unbiasedHSIC()
Double_t URANIE::Sensitivity::THSIC::unbiasedHSIC | ( | const TMatrixDSym & | matKx, |
const TMatrixDSym & | matKy | ||
) |
Computes the U-statistic estimate of \( HSIC(X, Y) \). The computation is based on the Gram matrices \( K_{x} \) and \( K_{y} \) which are constructed beforehand from the observations of \( X \) and \( Y \).
The formula of the U-statistic estimator can be found in: [Zhang et al., 2018] "Large-scale kernel methods for independence testing", Statistics and Computing", page 117, Eq (8).
Let \( L_{x} \) be the matrix obtained from the Gram matrix \( K_{x} \) after setting the diagonal elements to zero. Let \( L_{y} \) be the matrix obtained from the Gram matrix \( K_{y} \) after setting the diagonal elements to zero. Let \( 1 \) be the vector of \( \mathbb{R}^{nS} \) filled with ones.
The formula of the U-statistic estimator is given by:
\[ \widehat{HSIC}_{U}(X, Y) \, = \, \frac{1}{ nS (nS-3) } \, \left[ Tr( L_{x} L_{y} ) + \frac{1}{ (nS-1)(nS-2) } \, 1^T L_{x} 1 \times 1^T L_{y} 1 - \frac{2}{ nS-2 } \, 1^T L_{x} L_{y} 1 \right] \]
- Parameters
-
matKx (TMatrixDSym) The Gram matrix built from the observations of \( X \). matKy (TMatrixDSym) The Gram matrix built from the observations of \( Y \).
- Returns
- (Double_t) The value of the U-statistic estimate of \( HSIC(X, Y) \).
Referenced by ClassImp().
Member Data Documentation
◆ _nEstimatedVariance
|
private |
< The H matrix defined by H := ( Kronecker(i,j) - 1/nS)
Referenced by ClassImp(), and getEstimatedVariance().
◆ _nPermutationSample
|
private |
the method to compute the variance for the gaussian kernel
Referenced by ClassImp(), and getNPermutationSample().
◆ _nThresholdGamma
|
private |
The Threshold (nS/nX) to use the permutation permutation test to cumpute the p-value (default 5)
Referenced by ClassImp(), and getThresholdGamma().
◆ _nThresholdPermutation
|
private |
The number of permutation sample ( permutation ) (default NDefaultPermutation)
Referenced by ClassImp(), and getThresholdPermutation().